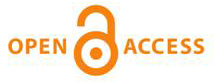
Address: Naberezhnaya Severnoy Dviny, 17, Arkhangelsk, 163002, Russian Federation, Northern (Arctic) Federal University named after M.V.Lomonosov, office 1425
Phone: +7 (8182) 21-61-18
E-mail: forest@narfu.ru
http://lesnoizhurnal.ru/en/
|
Identification of Damage to Coniferous Stands Based on Comprehensive Analysis of the Results of Remote Sensing of the Earth and Ground Surveys. P. 11–28
|
 |
Aleksandr S. Alekseev, Dmitriy M. Chernikhovskiy
UDС
630*5:528.85:630*9
DOI:
10.37482/0536-1036-2024-2-11-28
Abstract
Damage to forest stands and their death as a result of outbreaks of destructive insects and diseases occur over significant areas and represent an important forestry problem. To solve this problem, it is advisable to use materials from remote sensing of the Earth, since damaged and dead forests can occupy significant areas, from remote еach other. The article presents a methodology for assessing the state of forest stands and the impact of forest pests and diseases on them based on the joint processing of data from a regular grid of sample plots and materials from remote sensing of the Earth via geoinformational technologies, variance and regression analyses. The sample plots have been laid according to the ICPForests methodology and have characterized the general background of the state of the forests in the study area of the North-Western and central parts of the Leningrad Region, where the foci of forest pests and diseases reproduction have been identified at the same time. The applicability of 2 vegetation indices – normalized difference (NDVI) and short–wave (SWVI), as well as the normalized difference moisture index (NDMI), the normalized burn ratio (NBR) and the spectral characteristics of the corresponding channels of the Sentinel-2B image to identify the foci of forest damage by pests and diseases has been assessed. Statistically significant relationships between the state of the stands and the values of the listed indices have been established. It has been shown that the foci of pests and diseases reproduction are reliably detected using the materials from remote sensing of the Earth against the general background of the state of the forests represented by the regular grid of sample plots. A thematic map of the stands has been developed dividing them into 2 groups of state classes: healthy and weakened stands; suppressed stands and deadwood. Its accuracy was assessed via the support vector machine (SVM) on the basis of the combination of ground and remote sensing data. To assess the adequacy of the automatic classification, the error matrix and the calculation of the Cohen’s Kappa coefficient have been used. The coefficient has turned out equal to 0.878, which indicates a high quality of the classification. It has been shown that the developed thematic map is applicable for detecting potential foci of forest pests and diseases reproduction in the study area.
KeywordsICP-Forests, state classes, remote sensing methods, Sentinel-2B, automatic image classification, support vector machine (SVM), vegetation index, normalized difference moisture index (NDMI), normalized burn ratio (NBR), thematic map, the Leningrad Region
For citation
Alekseev A.S., Chernikhovskiy D.M. Identification of Damage to Coniferous Stands Based on Comprehensive Analysis of the Results of Remote Sensing of the Earth and Ground Surveys. Lesnoy Zhurnal = Russian Forestry Journal, 2024, no. 2, pp. 11–28. (In Russ.). https://doi.org/10.37482/0536-1036-2024-2-11-28
References
-
Akhmatovich N.A., Selikhovkin A.V., Magdeev N.G. Risk Management in the Republic of Tatarstan: Pests and Diseases of the Main Forest Forming Plants. Lesnoy Zhurnal = Russian Forestry Journal, 2015, no. 1, pp. 21–34. (In Russ.). https://doi.org/10.17238/issn0536-1036.2015.1.21
-
Aerospace Methods in Nature Conservation and Forestry. Ed. by V.I. Sukhikh, S.G. Sinitsyna. Moscow, Lesnaya promyshlennost’ Publ., 1979. 287 p. (In Russ.).
-
Bartalev S.A., Egorov V.A., Krylov A.M., Stytsenko F.V., Khovratovich T.S. The Evaluation of Possibilities to Assess Forest Burnt Severity Using Multi-Spectral Satellite Data. Sovremennye problemy distantsionnogo zondirovaniya Zemli iz kosmosa = Current Problems in Remote Sensing of the Earth from Space, 2010, vol. 7, no. 3, pp. 215–225. (In Russ.).
-
Bartalev S.A., Stytsenko F.V., Egorov V.A., Loupian E.A. Satellite-Based Asessment of Russian Forest Fire Mortality. Lesovedenie = Russian Journal of Forest Science, 2015, no. 2, pp. 83–94. (In Russ.).
-
Bartalev S.A., Stytsenko F.V., Khvostikov S.A., Loupian E.A. Methodology of Post-Fire Tree Mortality Monitoring and Prediction Using Remote Sensing Data. Sovremennye problemy distantsionnogo zondirovaniya Zemli iz kosmosa = Current Problems in Remote Sensing of the Earth from Space, 2017, vol. 14, no. 6, pp. 176–193. (In Russ.). https://doi.org/10.21046/2070-7401-2017-14-6-176-193
-
Vorobiev O.N., Kurbanov E.A., Demisheva E.N., Menshikov S.A., Ali M.C., Smirnova L.N., Tarasova L.V. Remote Monitoring of Forest Ecosystems Sustainability. Ed. by prof. E.A. Kurbanov. Yoshkar-Ola, Volga State University of Technology, 2019. 166 p. (In Russ.).
-
Grigoryeva O.V. Observation of Forest Degradation Using Hyperspectral Data Aerial and Satellite Sensing. Earth Observation and Remote Sensing, 2014, no. 1, pp. 43–48. (In Russ.). https://doi.org/10.7868/S020596141306002X
-
Karpov A., Waske B. Method for Transferring Non-Forest Cover to Forest Cover Land Using Landsat Imageries. Lesnoy Zhurnal = Russian Forestry Journal, 2020, no. 3, pp. 83–92. (In Russ.). https://doi.org/10.37482/0536-1036-2020-3-83-92
-
Knyazeva S.V., Eydlina S.P. Cartographic Estimation of Tree Parameter Dynamics in Russian Northwest Regions. Voprosy lesnoy nauki = Forest Science Issues, 2018, vol. 1(1), pp. 1–33. (In Russ.). https://doi.org/10.31509/2658-607X-2018-1-1-1-33
-
Kovalev A.V. Analysis of Forest Stands Resistance to Siberian Silkmoth Attack According to Remote Sensing Data. Sibirskiy lesnoy zhurnal = Siberian Journal of Forest Science, 2021, no. 5, pp. 71–78. (In Russ.). https://doi.org/10.15372/SJFS20210508
-
Krylov A.M., Sobolev A.A., Vladimirova N.А. Revealing of Centers Ips typographus in Moscow Region with Use of Pictures Landsat. Lesnoy vestnik = Forestry Bulletin, 2011, no. 4, pp. 54–60. (In Russ.).
-
Malakhova E.G., Krylov A.M. The Fir Groves Drying in Klinsky Forestry of Moscow Oblast. Izvestia of Samara Scientific Center of the Russian Academy of Sciences, 2012, vol. 14, no. 1(8), pp. 1975–1978. (In Russ.).
-
Methods for Monitoring Forest Pests and Diseases. Ed. by V.K. Tuzov. Moscow, VNIILM Publ., 2004. 200 p. (In Russ.).
-
Silkina O.V., Vinokurova R.I. Seasonal Dynamics of Chlorophyll and Microelement Content in Developing Conifer Needles of Abies sibirica and Picea abies. Fisiologiya Rasteniy = Russian Journal of Plant Physiology, 2009, vol. 56, no. 6, pp. 864–870. (In Russ.).
-
Stytsenko F.V., Bartalev S.A., Bukas A.V., Ershov D.V., Saigin I.A. The Possibilities of Prolonged Burnt Severity Assessment of Evergreen Coniferous Forest Using Multi-Spectral Satellite Data. Sovremennye problemy distantsionnogo zondirovaniya Zemli iz kosmosa = Current Problems in Remote Sensing of the Earth from Space, 2019, vol. 16, no. 5, pp. 217–227. (In Russ.). https://doi.org/10.21046/2070-7401-2019-16-5-217-227
-
Sukhikh V.I. Aerospace Methods in Forestry and Landscape Construction. Yoshkar-Ola, MarSTU Publ., 2005. 392 p. (In Russ.).
-
Tokareva O.S. Processing and Interpretation of the Earth Remote Sensing Data. Tomsk, TPU Publ., 2010. 148 p. (In Russ.).
-
Fedotova E.V., Zarechneva A.I. Spatial-Temporal Dinamics of Siberian Silkmoth Outbreak in Dark Needle Coniferous Forest in Altay Mountains. Zhurnal Sibirskogo federal’nogo universiteta. Tekhnika i tekhnologii = Journal of Siberian Federal University. Engineering & Technologies, 2017, vol. 10, no. 6, pp. 747–757. (In Russ.). https://doi.org/10.17516/1999-494X-2017-10-6-747-757
-
Khumala A.E., Polevoy A.V., Shalaev V.S., Galkin Yu.S., Shcherbakov A.N., Naldeev D.F., Nikula A., Rojninen H. Assessment of the Possibility of Using Aerospace Methods for Monitoring Forest Cover in the «Vodlozersky» National Park. Vodlozersky Readings: Natural Science and Humanitarian Foundoctions of Environmental, Scientific and Educational Activities in Protected Natural Areas of the Russian North: Materials of the Scientific and Practical Conference Dedicated to the 15th Anniversary of the “Vodlozersky” National Park (Petrozavodsk, April 27–28, 2006). Petrozavodsk, Karelian Research Centre Publ., 2006, pp. 106–112. (In Russ.).
-
Cherepanov A., Druzhinina E. Spectral Characteristics of Vegetation and Vegetation Indexes. Geomatica = Geomatics, 2009, no. 3, pp. 28–32. (In Russ.).
-
Shatalov A.V., Zhirin V.M., Sukhikh V.I., Eydlina S.P., Shalaev V.S. To the Assessment of Damage to the Forests of the «Vodlozersky» National Park Based on Satellite Images. Natural Science and Humanitarian Foundoctions of Environmental, Scientific and Educational Activities in Protected Natural Areas of the Russian North. Materials of the Scientific and Practical Conference Dedicated to the 15th Anniversary of the “Vodlozersky” National Park (Petrozavodsk, April 27–28, 2006). Petrozavodsk, Karelian Research Centre Publ., 2006, pp. 102–106. (In Russ.).
-
Shelukho V.P., Shoshin V.I., Klyuev V.S. Sanitary State Dynamics of Spruce Forests Under Culminating Ips typographus Reproduction and Efficiency of Forest-Protection Measures. Lesnoy Zhurnal = Russian Forestry Journal, 2014, no. 2, pp. 30–39. (In Russ.).
-
Alekseev A., Chernikhovskii D. Assessment of the Health Status of Tree Stands Based on Sentinel - 2B Remote Sensing Materials and the Short-Wave Vegetation Index SWVI. IOP Conference Series: Earth and Environmental Science, 2021, vol. 876, art. no. 012003. https://doi.org/10.1088/1755-1315/876/1/012003
-
Alekseev A., Chernikhovskii D., Vetrov L., Gurjanov M., Nikiforchin I. Determination of the State of Forests Based on a Regular Grid of Ground-Based Sample Plots and Sentinel-2B Satellite Imagery Using the k-NN («Nearest Neighbour») Method. IOP Conference Series: Earth and Environmental Science, 2021, vol. 876, art. no. 012002. https://doi.org/10.1088/1755-1315/876/1/012002
-
Congalton R.G., Green K. Assessing the Accuracy of Remotely Sensed Data. Principles and Practices. 3rd ed. Boca Raton, CRC Press, 2019. 346 p. https://doi.org/10.1201/9780429052729
-
Conrad O., Bechtel B., Bock M., Dietrich H., Fischer E., Gerlitz L., Wehberg J., Wichmann V., Böhner J. System for Automated Geoscientific Analyses (SAGA) v. 2.1.4. Geoscientific Model Development, 2015, vol. 8, iss. 7, pp. 1991–2007. https://doi.org/10.5194/gmd-8-1991-2015
-
Fan R.-E., Chen P.-H., Lin C.-J. Working Set Selection Using Second Order Information for Training Support Vector Machines. Journal of Machine Learning Research, 2005, vol. 6, pp. 1889–1918.
-
Fisher R., Hobgen S., Mandaya I., Kaho N., Zulkarnain N. Satellite Image Analysis and Terrain Modelling. A Practical Manual for Natural Resource Management, Disaster Risk and Development Planning Using Free Geospatial Data and Software. Version 2. SAGA GIS 4. Charles Darwin University, 2017. 150 p.
-
Franklin S.E. Remote Sensing for Sustainable Forest Management. Boca Raton, CRC Press, 2001. 424 p. https://doi.org/10.1201/9781420032857
-
Kharuk V.I., Ranson K.J., Kozuhovskaya A.G., Kondakov Y.P., Pestunov I.A. NOAA/AVHRR Satellite Detection of Siberian Silkmoth Outbreaks in Eastern Siberia. International Journal of Remote Sensing, 2004, vol. 25, iss. 24, pp. 5543–5556. https://doi.org/10.1080/01431160410001719858
-
Hsu C.-W., Chang C.-C., Lin C.-J. A Practical Guide to Support Vector Classification, 2016. 16 p.
-
Lillesand T.M., Kiefer R.W., Chipman J.W. Remote Sensing and Image Interpretation. 7th ed. New York, Wiley Inc., 2015. 736 p.
-
Lukeš P. Monitoring of Bark Beetle Forest Damages. Big Data in Bioeconomy. Springer Publ., 2021, chapt. 26, pp. 351–361. https://doi.org/10.1007/978-3-030-71069-9_26
-
Nelson R.F. Detecting Forest Canopy Change Due to Insect Activity Using Landsat MSS. Photogrammetric Engineering and Remote Sensing, 1983, vol. 49, no. 9, pp. 1303–1314.
-
Olsson P.-O., Kantola T., Lyytikäinen-Saarenmaa P., Jönsson A.M., Eklundh L. Development of a Method for Monitoring of Insect Induced Forest Defoliation – Limitation of MODIS Data in Fennoscandian Forest Landscapes. Silva Fennica, 2016, vol. 50, no. 2, art. no. 1495. https://doi.org/10.14214/sf.1495
-
Olsson P.-O., Lindström J., Eklundh L. Near Real–Time Monitoring of Insect Induced Defoliation in Subalpine Birch Forests with MODIS derived NDVI. Remote Sensing of Environment, 2016, vol. 181, pp. 42–53. https://doi.org/10.1016/j.rse.2016.03.040
-
QGIS and Applications in Agriculture and Forest. Vol. 2. Ed. by N. Baghdadi, C. Mallet, M. Zribi. Wiley Inc., 2018. 368 p.
-
Zhirin V.M., Knyazeva S.V., Eydlina S.P. Dynamics of Spectral Brightness of the Species/Age Structure for Groups of Forest Types on Landsat Satellite Images. Contemporary Problems of Ecology, 2014, vol. 7, pp. 788–796. https://doi.org/10.1134/S1995425514070142
-
Zhirin V.M., Knyazeva S.V., Eydlina S.P. Long-Term Dynamics of Vegetation Indices in Dark Coniferous Forest after Siberian Moth Disturbance. Contemporary Problems of Ecology, 2016, vol. 9, pp. 834–843. https://doi.org/10.1134/S1995425516070118
|
Make a Submission
Lesnoy Zhurnal (Russian Forestry Journal) was awarded the "Seal of Recognition for Active Data Provider of the Year 2024"
|